GeoMatch: A Flexible and Effective Matching Technology
GeoMatch is a flexible technology platform designed to to help match people to places where they are most likely to thrive. It can function as a standalone web application or be seamlessly integrated into existing IT systems, adapting to your organization’s workflow.
Flexible and Customizable
GeoMatch is designed to enhance existing workflows, presenting critical information in a clear, easy-to-understand format. Partners can customize the platform to prioritize factors such as maximizing outcomes, ensuring balanced distribution, or incorporating user preferences. This flexibility ensures GeoMatch can meet diverse goals while remaining rooted in data-driven insights.
At its core, GeoMatch combines advanced analytics with human insight through the following key elements:
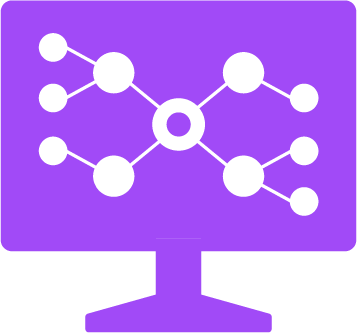
Modeling Machine learning uncovers patterns in historical data, revealing how different people succeed in various locations.
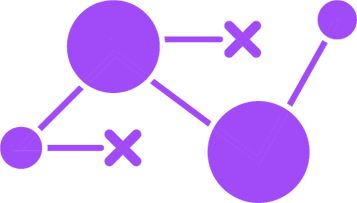
Predictions These patterns are transformed into personalized predictions for each individual's potential success across locations.
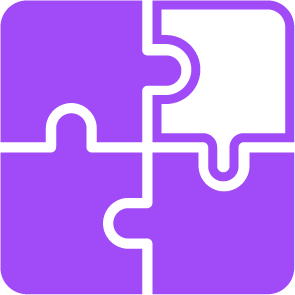
Matching GeoMatch identifies optimal matches by balancing predictions, preferences, and real-world constraints like capacity and services.
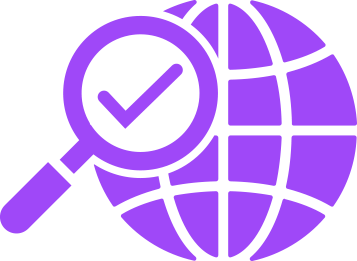
Recommendations GeoMatch delivers clear, actionable insights through a user-friendly interface, empowering users to make more informed decisions.
Framework
GeoMatch’s Approach to Ethical AI
We believe technology can help solve complex social challenges, but it needs to be developed thoughtfully and responsibly.
Outcome-based matching isn’t limited to immigration. Explore how GeoMatch can work for you:
- Refugee resettlement and immigration services
- Workforce optimization
- Education placement
- Community service coordination
- Housing solutions
Get in touch with GeoMatch Director Michael Hotard to learn how GeoMatch can fit your context.
The Research Behind GeoMatch
We are committed to generating high-quality evidence of the benefits possible from using GeoMatch. Our initial research showed how existing historical data could be leveraged to improve economic outcomes for refugees and asylum seekers by identifying better resettlement destinations.
Current research builds on this finding, addressing other dimensions of human-centered AI and matching. These include incorporating immigrant preferences, making sure allocations are balanced across locations, and ensuring recommendations don’t benefit some groups at the expense of others. Future research will address challenges of how to use AI-driven tools in the public sector and provide evidence of actual gains in refugee and immigrant integration from using GeoMatch.
Publications
Improving refugee integration through data-driven algorithmic assignment
2018 | Science | Kirk Bansak, Jeremy Ferwerda, Jens Hainmueller, Andrea Dillon, Dominik Hangartner, Duncan Lawrence, Jeremy Weinstein
The authors developed an algorithm that incorporates machine learning to place refugees in locations that optimize their employment. Tests using historical data demonstrated the algorithm improved the employment prospects of refugees in the United States by 40% and in Switzerland by 75%.
Combining outcome-based and preference-based matching: a constrained priority mechanism
2021 | Political Analysis | Avidit Acharya, Kirk Bansak, Jens Hainmueller
The authors developed a mechanism that enables the GeoMatch algorithm to incorporate user preferences. The authors demonstrate how the mechanism could assist with two use cases: the assignment of refugee families to host country locations and kindergarteners to schools. This adjustment to the algorithm improves the quality of its recommendations by ensuring they incorporate individual preferences while still improving social welfare.
Outcome-Driven Dynamic Refugee Assignment with Allocation Balancing
2024 | Operations Research | Kirk Bansak, Elisabeth Paulson
The authors propose and test two algorithms to match refugees and asylum seekers to locations within a host country. The first seeks to maximize employment levels (or any outcome of interest) for refugees, and the second balances the goal of improving refugee outcomes with the desire for an even allocation across host sites.Test results show very similar employment-maximization for both algorithms, with much better geographic balance for the second, ensuring no single placement site is over- or under- used.
Learning Under Random Distributional Shifts
2024 | Proceedings of Machine Learning Research | Kirk Bansak, Elisabeth Paulson, Dominik Rothenhaeusler
The authors explored different methods for making predictions in situations where the demographic characteristics of refugees and economic characteristics of locations change over time. They considered three methods: the standard approach of using data on past arrivals to predict the (often long-term) policy outcome of interest; an approach that uses newer data on proxy outcomes; and a hybrid approach that leverages both the policy outcome and more recent proxy outcomes. They found that the hybrid approach performed best when tested using data from asylum-seeker placement and early childhood education.
Working Papers
Leveraging the Power of Place: A Data-Driven Decision Helper to Improve the Location Decisions of Economic Immigrants
2020 | Jeremy Ferwerda, Nicholas Adams-Cohen, Kirk Bansak, Jennifer Fei, Duncan Lawrence, Jeremy M. Weinstein, and Jens Hainmueller
The authors propose a version of the GeoMatch tool that would act as a "decision helper" for economic immigrants coming into Canada. The tool would provide personalized location recommendations that reflect immigrants' preferences as well as data-driven predictions of the locations where they would be able to maximize their expected earnings. Simulations using data from recent economic immigrants in Canada suggest these recommendations can increase initial earnings and lead to a mild shift away from the most populous landing destinations.
Group fairness in dynamic refugee assignment
2023 | Daniel Freund, Thodoris Lykouris, Elisabeth Paulson, Bradley Sturt, Wentao Weng
The authors developed a framework for incorporating group fairness into refugee placement so that the gains are more evenly distributed across groups (e.g., refugees with different education levels or countries of origin). Using this framework, the authors test two bid-price algorithms using various definitions of group fairness and real-world data from the U.S. and the Netherlands. These experiments demonstrate the algorithms can improve group fairness with only small decreases in global performance.
Dynamic Matching with Post-Allocation Service and its Application to Refugee Resettlement
2024 | Kirk Bansak, Soonbong Lee, Vahideh Manshadi, Rad Niazadeh, Elisabeth Paulson
The authors developed a new learning-based algorithm for dynamic matching that could be used for improving matches between refugees and their locations. The new algorithm eliminates the need for assumptions on the future distribution of refugees that current approaches rely on. When tested on historical data, the new algorithm outperformed existing algorithms used for refugee matching.